Research and Development
Confronted with the operational use of AI through its customers, Mangrove knows perfectly well the limits of current industrial solutions. This is why we have been investing in Research since our beginnings to solve the most critical problems through close links with the scientific community.
A common problem that affects all industries and that will grow with the rise of AI is the drift of learning models: detection, on-the-fly updating and correction of drift.
Indeed, an AI/ML model is static and undergoes a degradation (drift) of performance more or less quickly depending on various parameters. Continuous learning schemes exist but are often cumbersome and expensive to implement, which makes them not very operational. Drift detection tools can also be integrated in certain specific use cases, but most often require specific know-how in the field of application and a long adjustment of the parameters. Finally, models built around drift detection and correction are rare and offer uneven performance while often relying on unrealistic assumptions in a production environment.
After researching on data pipelines and the explicability of AI results, Mangrove is focusing its R&D efforts on drift management and operational constraints in partnership with the Laboratoire de Recherche en Informatique de l'Université de Bordeaux (LaBri) and some of our customers.
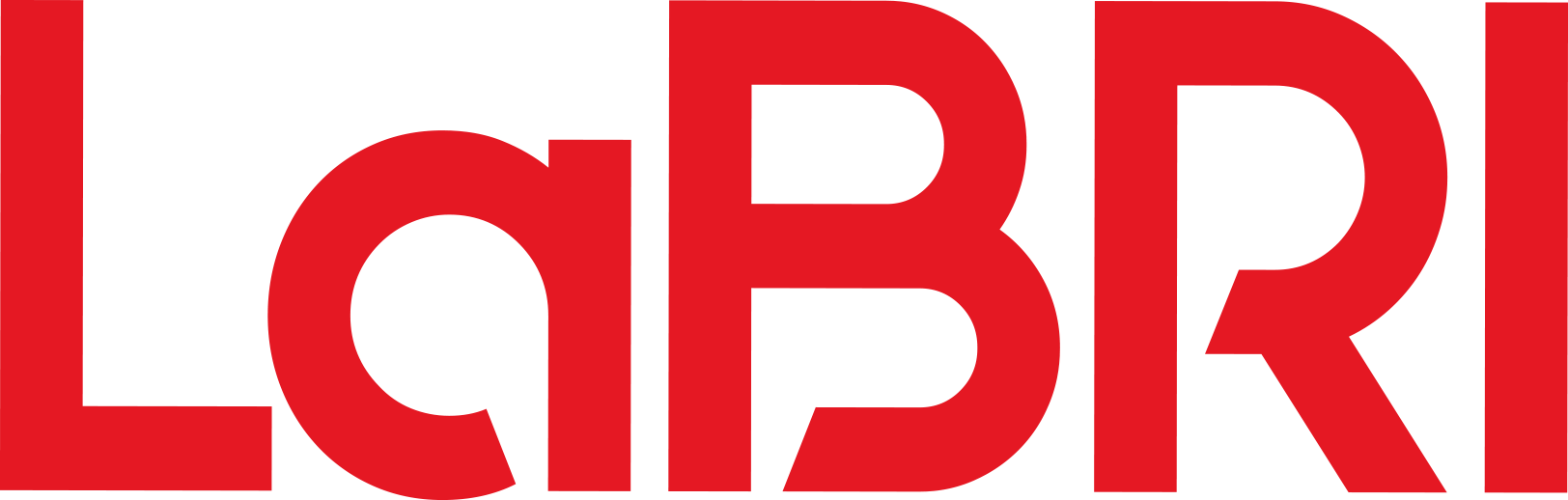
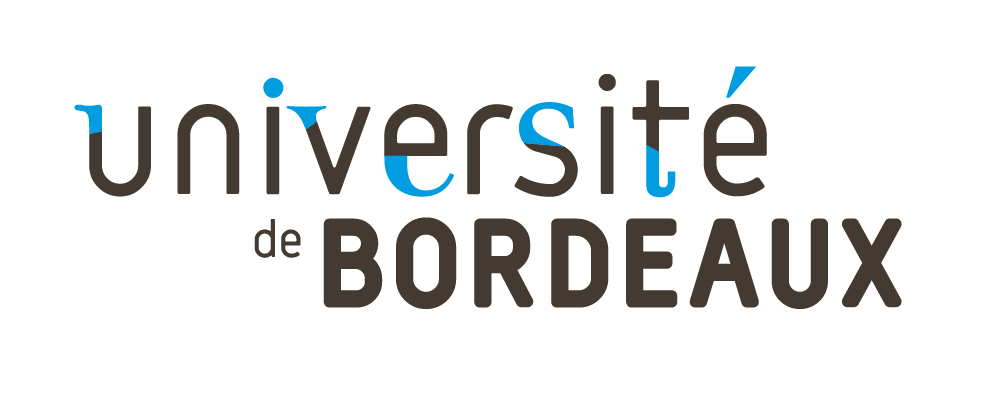
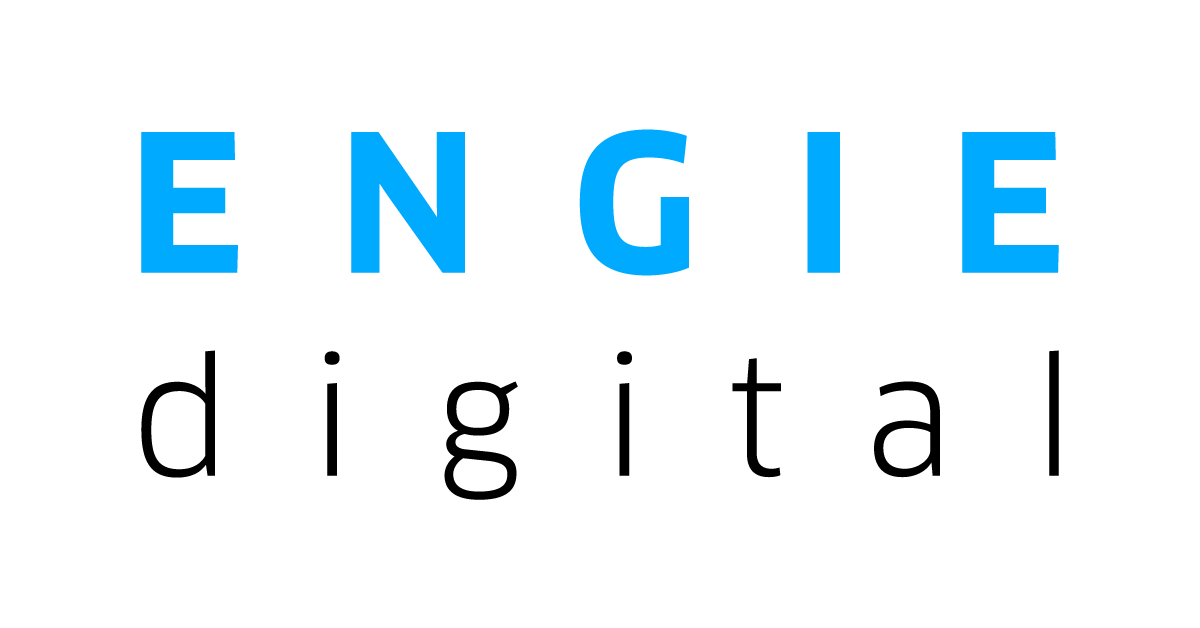